Machine learning has the potential to revolutionize the admissions process for graduate schools by providing a more objective and efficient way to evaluate candidates. Here are some ways in which machine learning could change the admissions process for graduate school:
- Bias Reduction: Machine learning algorithms can be trained to reduce bias in the admissions process by removing identifying information such as names and addresses from application materials, and evaluating candidates solely on their qualifications and fit for the program.
- Predictive Modeling: Machine learning models can analyze historical data on successful candidates and use that information to predict the likelihood of a candidate’s success in the program. This could help admissions officers identify high-potential candidates who may not have met traditional criteria, such as GPA or standardized test scores.
- Personalized Recommendations: Machine learning algorithms can analyze the strengths and weaknesses of each applicant and provide personalized recommendations for how they could improve their chances of being accepted. This could help candidates better understand the admissions process and make more informed decisions about their application.
Machine learning could help make the graduate school admissions process more objective, efficient, and fair, while also providing candidates with more personalized feedback on their applications. However, it’s important to note that machine learning models are only as good as the data they are trained on, so it’s crucial to ensure that the data used is diverse, representative, and unbiased.
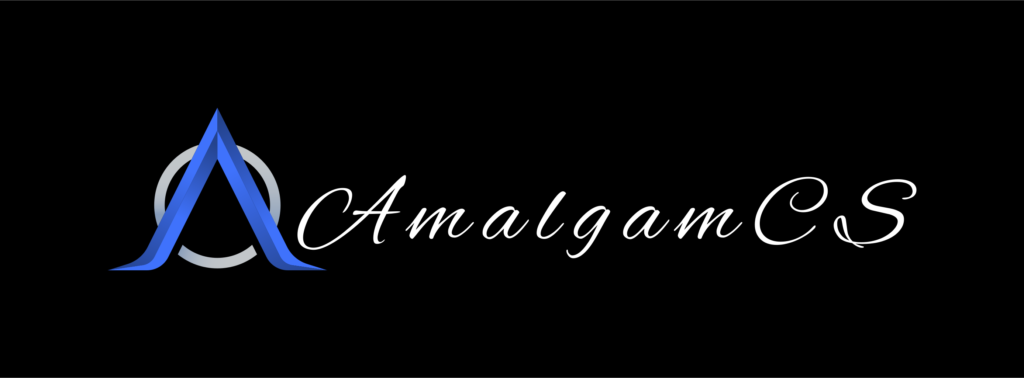